- Wednesday, 19 Nov 2008:
The Open Rights Group
- Sunday, 21 Sep 2008:
Interface magic
- Thursday, 11 Sep 2008:
Improvising makes me gulp
- Tuesday, 2 Sep 2008:
Thoughts on Google Chrome
- Tuesday, 24 Jun 2008:
Complexity increases
- Tuesday, 24 Jun 2008:
Plain Scaling
- Tuesday, 17 Jun 2008:
Widefinder: Final Results
- Thursday, 12 Jun 2008:
Widefinder: Paying Attention
- Tuesday, 10 Jun 2008:
Widefinder: Interesting Ways To Suck
- Monday, 9 Jun 2008:
Thoughts on Widefinder
- Published at
- Wednesday 19th November, 2008
- Tagged as
The Open Rights Group (sometimes known by their un-Googlable acronym ORG) has turned three. Happy birthday, and congratulations!
To, erm, me. When the initial word went out in mid 2005, I pledged to become a founder member, and (beyond a short period where PayPal cancelled my subscription) I've been a supporter ever since. Not a particularly active one, admittedly.
Anyway, as you can see from their birthday review, they've achieved lots of really good things - getting the word out on digital rights issues, working with policy makers, and confronting police officers using clipboards and hand gestures. These kinds of challenges aren't going away, so please help out, or give financial support, or even both.
- Published at
- Sunday 21st September, 2008
- Tagged as
- Interfaces
- Interaction
- UI
- Doom
The PlayStation 3 has a pretty good interface for playing DVDs, all things told: the key controls are immediately available from the controller, mostly using shoulder buttons as jogs, and I'd guess that most people who have got a PS3 and know what a DVD is have little difficulty using it. The problem arises with the magic.
Magic interfaces are one of those things that are a very good idea, but very difficulty to get exactly right: you want to take the thinking away from the user, so that stuff just works, but this means that if there's any significant mismatch between what you think people will want to happen and what they actually want, your users will start getting frustrated.
This is the area that Apple lives in, at least for the iPod and iPhone: don't think about how this thing works, just assume it will, and get stuck into it. By and large they do pretty well, and by and large Sony do okay with the PS3 also. The key magic that Sony have come up with (or at least the bit that I've noticed) is around what happens when you don't finish watching a film, but need to take the disc out. This is a pretty common requirement with a games machine, so it's not difficult to see why they've decided to make this simple: put the disc back in, and the PS3 will pick up where you left off.
This would be an unambiguously good thing, except for a couple of points. Firstly, and less importantly, if you eject the disc at the end of the movie (or TV episode, or whatever), while the credits are running, then the next time you put the disc in it'll jump back to that point, and you have to navigate back to the menu - which some DVDs make much harder than others, because of their desire to show lots of copyright notices for countries I'm not resident in. (I suspect this doesn't happen in the US, and perhaps also not in Japan either.) If DVD authors would stop trying to persuade us that we're criminals, this would be a non-issue.
But the second issue was much more of a pain when I encountered it. Something I didn't notice for ages. Something which is almost never important, because it's simply not something you're likely to want to do.
I started watching an episode of something, and noticed there was a commentary by the writer, which I thought might be interesting... and after about five minutes decided it wasn't. So I ducked out of playback back to the menu, and hit the 'play episode' button instead of the 'play with commentary'. And the PS3 helpfully picked up where I'd stopped, with the commentary track.
It took me perhaps ten minutes of turning the machine off and back on, ejecting the disc, and so forth, until I figured out that I could turn the commentary off by resetting the language options on the disc. (For some reason the audio tracks control was disabled for the disc.)
The question, of course, is: is this remotely important? I've been playing DVDs on the PS3 for about eight months, and I haven't run into this problem before now. Most people, I'd guess, don't listen to commentaries anyway; those that do probably only seldom back out once they've started. And most DVDs probably won't cause this problem, because they won't have the audio tracks disabled. So it isn't actually important at all.
The important point is that magic is by its nature opaque; if it weren't, it wouldn't be magic. And, like diesel engines and anything containing a class 4 laser, you can't take apart magic and figure out what isn't working. Instead, you have to build up a conceptual model of how it works inside, and figure out how to game it - which is doubly difficult because the point where the magic needs fixing is the point where the conceptual model that you already have doesn't match the magic in the first place. All your preconceptions go out of the window, and you have to think. Uh-oh.
There are two solutions to this when designing a system. One is not to care: this is the simple route, and has many advantages (including simpler testing). The other is to provide a way of turning the magic off; a kind of less magic switch. Personally I think that the former is a better choice: decide how your system will work, and trust to your own ability to make good decisions. Of course, you may get feedback that suggests you're better off removing the magic entirely, but options force people to think, which goes against the reason you wanted to introduce the magic in the first place.
Just use the best magic you can possibly manage.
- Published at
- Thursday 11th September, 2008
- Tagged as
- Improvisation
- Teaching
- Terror
- Seedcamp
- Mentoring
Improvising makes me gulp; I get a visceral reaction before I go on stage. To be honest, this is a reaction I have to lots of similar situations: concerts where I was a soloist while back at school, plays, presenting, teaching. It's part of why I do it, although it doesn't feel like that at the time: adrenaline starts to rush through my body, and I want to throw up. But that passes, quickly, and then I'm on a high. I could probably sit down and rank the different things I do according to how great I feel doing them, and afterwards. Improvisation, and teaching improvisation, would come out at the top.
For the last few Barcamps I've been to I've taught impro for half an hour to whoever turns up; Barcamp Brighton 3, last weekend, was no exception. Best of all, we had a big enough crowd to play one of my favourite games to wrap up. I know it as The King Game, from Keith Johnstone (p237 of the Faber & Faber edition of Impro for Storytellers, if you have it), and it's particularly satisfying because you end up with a huge mound of bodies on the stage, all of whom are still paying attention to the scene.
Basically, people come in as servants, and the King orders them to commit suicide when (as inevitably happens) they get irritated. It's actually very hard to be a good servant, but some people actually try very hard to be bad (and in a quick session, it's generally more satisfying to play like this, admittedly breaking all the rules of good impro). Where it gets interesting is where people come up with strategies to avoid being ordered to die; at the weekend, someone came on as the King's daughter. (I think she actually lasted less time than the average.) The only time I've ever seen someone come on and survive was when I was doing this in Cambridge, preparing for a Whose Line Is It Anyway-style late-night show, and one of the women walked on and seduced the King. I suspect that works quite well as a strategy in real life, as well.
I've actually done much more of teaching improvisation than performing over the last couple of years (something I hope to change); but it does at least provide a (weak) segue to Seedcamp, where I'm a technical mentor this year. Looking over the entire list of mentors is a little daunting, but there are enough people on the list that I know from various things to make me feel I'll fit in. If you're one of this year's 22 finalists, I'll see you on Wednesday 17th, talking about How to Scale. According to Tom Coates, it has something to do with elephants.
- Published at
- Tuesday 2nd September, 2008
- Tagged as
- Google
- Web browser
- Unwise predictions
First, let's start by saying that having a new web browser on the market to shake things up is never going to be a bad thing; and having something fresh from Ben Goodger is always going to be fun. The other people on the Chrome team sound smart as well, and it's clear that they're trying to solve a problem using a savvy blend of UI nous, applied research, and good software development. All that stuff about testing? Awesome. Oh, and using Scott McCloud for your promo literature is inspired.
But... am I really the only person who disagrees with their fundamental tenet? They claim that the majority of people's use of the web is as applications, not as web pages. I'm sure this is true of people inside Google (if nothing else because it can cause problems when they don't have high uptime), but I'm less than convinced for the general populace. It's certainly not true of me: of the tabs I have open in my browser right now, only seven fall within their broad definition of 'web applications' (actually three are films and one a list of audio clips, both of which I'd actually have watched or listened to by now if they'd instead been presented as something I could shunt onto my iPod; one is the Google Chrome comic itself, which I include as a web application mostly to deride the fact that it requires Javascript to function for absolutely no reason, giving absolutely no benefit to the user), compared to 41 'normal' pages (six items on shopping sites which use little or no Javascript; most of the rest are articles, main pages of sites with lots of articles, blogs or software sites). My remaining two tabs are one site that's down (and I can't remember what it is), and MySpace (which is anybody's guess how to classify). That's around 16% 'web applications', or a mere 6% if people would have done things properly in the first place.
Okay, so - disclaimers. I don't use web mail, which would definitely be an application, and would probably count for a reasonable amount of my usage online if I used it. I do use Facebook, sometimes, but I don't have it open anywhere right now; in fact, I almost never leave Facebook open, except for event information, which in my book is a web page not a web application. However I'm perfectly prepared to admit that I might be unusual. Freakish, even.
Of course, I'll benefit from a faster Javascript engine once they release on the Mac (on Windows I run Firefox with NoScript, so frankly I couldn't care one way or the other); and the process separation of tabs is smart (and, unlike others who've thought of it in the past, they've actually gone to the effort of doing it). But what I really want is genuine, resource-focussed, web-browsing features. Like, I don't know, proper video and audio support in the browser.
What's that you say, Lassie? Little Timmy's done what?.
However it's a huge deal to bring a new browser to market (or even to beta), so congratulations to the Google Chrome team (although... Chrome... really?). As they say, it's open source, so everyone can learn from each other. (Although of course strictly speaking this is Chris diBona wafflecrap, but that's for another post entirely.) But I'm not convinced this is on the critical path between us and jetpacks. Not even little ones.
- Published at
- Tuesday 24th June, 2008
Or more accurately: complexity never decreases, both in the strict sense that if something’s O(n)
, it will always be O(n)
, and if you find a faster way of doing it, the complexity hasn’t changed, you’ve just been really smart (or you were particularly dumb to start off with).
But I also mean this in another way, with a more sloppy use of the word ‘complexity’: that just because you’ve done something before doesn’t necessarily mean it will be easier this time round. The complexity of the problem is the same, after all; the only real advantage you’ve got from having done it before is an existence proof. I think this is an important point that a lot of people miss. Then they act all surprised when building a new widget takes longer than they expected.
Complexity never decreases. You can represent this mathematically as:
which is what I’ve got on my t-shirt today at the second half of the O’Reilly Velocity Conference, which is all about performance and operations for internet-scale websites. And there’s some good stuff here - besides interesting conversations with vendors, and a couple of product launches, the very fact that this is happening, rather than being confined to what I’m sure a lot of my fellow delegates consider more “academic” arenas such as Usenix feels like a positive step forward.
Of course, some of this isn’t new. One of the conference chairs is Steve Souders, who has been banging the drum about front-end web performance techniques for a while, so it’s not surprising that we’re seeing a lot of that kind of approach being talked about. Since I follow this stuff pretty closely anyway, even over the last six months when I’ve been only flakily connected to the industry, I know much of this already; however it doesn’t mean it’s a bad thing: there will be people here who haven’t had it explained sufficiently for them yet, so they’ll go away with important new tools for improving the sites they work on.
Some of the things people are saying are older yet; and some are a bad sign. At least four speakers yesterday laid into the advertising technology industry, including Souders. However I note that they don’t appear to have actually sat down with the tech vendors, and they haven’t got any representatives speaking here. No matter what people think, performance problems related to putting ads on your pages aren’t always the fault of the tech vendors, and even when they are they’re open and often eager to talk to people about improving things. There’s a panel this afternoon on how to avoid performance problems with adverts, which I’m sure will have some interesting and useful techniques, but I’m equally sure some of them will date very rapidly, and very few if any will have been submitted to the tech vendors to make sure that they don’t have unintended side-effects. People are thinking about this, which is good; but they also have to talk about it, and not just at smallish conferences in San Francisco, but at things like Ad:Tech in New York. Hopefully this is the start of the right approach, though: advertising isn’t going away.
I’ll probably have more thoughts by the end of the day, but for now sessions are starting up again, so I’m going to go learn.
- Published at
- Tuesday 24th June, 2008
- Tagged as
Update: Yahoo! Pipes is no more, and I never wrote much on Plain
Scaling, so I’ve folded the entries in here and shut it down.
The recent stuff on Wide Finder has been pretty fun, and I’m typing
this during the Velocity
conference on performance and
operations, so it seems as good a time as any to announce a new blog
I’ve created: Plain Scaling. I can’t
guarantee it’ll always have lots of stuff in there, but my thoughts on
scaling and performance will end up there. In particular, I’ve likely
got some more stuff on WF-2 coming following conversations with some
Sun guys at Velocity.
Of course, this is more of a pain to follow than just one blog, so
I’ve set up a little thing using Yahoo!
Pipes to bring them all into one RSS feed
containing all my blog
entries - at
least in theory. Currently it won’t cope with the Atom feed generated
from this blog, which seems to be a bug in Pipes; there’s definitely a
blog post coming on that. If I can’t fix it using Pipes, I’ll write my
own approach, but I really don’t want to.
Anyway. There you are.
- Published at
- Tuesday 17th June, 2008
- Tagged as
- Scaling
- Widefinder
- Parallelism
By the end of my last chunk of work on WideFinder, I'd found a set of things to investigate to improve performance. All were helpful, one way or another.
- Use fixed string searches either instead of (for referrer checking) or as well as (for page detection) regular expressions [Fredrik Lundh]
- Pre-fetch the regular expression method outside the loop [Fredrik Lundh]
- Snap my Python wrapper of
dict
to use a built-in [me]
- Use temporary files instead of pipes to communicate between workers and master [Alex Morega]
- Out of order processing [me]
- Reducing concurrent memory usage to fit within core [me]
- Use the CoolStack SPARC-optimised build of Python 2.5 [Tim Bray]
With smaller data sets, the first three together gave around a 10% improvement running on my dev machine; the last gave about 1.5% on the WF-2 machine over the 2.5 build I'd made myself; however I can't use it for the full run because it's 32 bit (the problem here is discussed below).
Initially I was running tests on my dev machine, using small amounts of data. There, out of order processing lost out slightly. It's bound to be a little slower in pure terms, because I had to use files instead of pipes for communication (I'm guessing the output was big enough to fill up the pipe; with some thought this could be fixed), but is more efficient with lots of workers across a large amount of data, because the run time variance increases. Additionally, the naive way of decreasing concurrent memory usage meant increasing the number of reduces; the alternative would increase the complexity of the user code, which I wanted to avoid. So there's probably some further improvements that can be made.
Anyway: my final result. I re-tested my two final versions, one with out-of-order processing and one not, on the 10m data sets before letting them both rip on the full data set. On the 10m line set, I was seeing up to a 25% improvement over my previous best run; for the whole lot, I saw only an 18% improvement: 34m15.76s. At this kind of scale, out of order processing, even with the file serialisation overhead, gives around a 10% speed improvement. (This is somewhat less than Mauricio Fernandez found doing pretty much the same thing in OCaml.)
This isn't even as good as the other Python implementation; for some reason I'm still getting nothing like efficient use out of the processor. Possibly having readers feeding the data to the processors over pipes might improve things here. I also still had to use the 64 bit version of Python, as Python's mmap
doesn't support the offset
parameter (there's a patch to allow it, which is private on the Python SourceForge site, sigh). In fact, it feels like every architectural optimisation I've tried to make has made things slower because of Python; the only things that have really speeded it up (beyond the initial parallelism) are the lower level optimisations that make the code less idiomatically Pythonic anyway.
It's possible that with some more thought and work, it would be possible to reduce this further; but I'd bet that Python will never get a run time below 15 minutes on WF-2 (although that would still put it in the same ballpark as the JVM-based implementations). At this point I'm mostly into optimising without being able to do the optimisations I want to, which seems fairly pointless; I've also sunk more time into this than I originally intended, so I'm going to stop there.
The code
User code (86 LOC)
I suspect that more code can be trimmed from here, particularly from the top()
function, but it seems neater in many ways to leave it largely as the direct translation of Tim's original, with a few optimisations. Python is somewhat more verbose than Ruby; for this kind of job I find the readability about the same.
The only optimisation I'm unhappy with is the line that tests for a fixed string and then tests for a regular expression that starts with that fixed string; this does seem to work faster, but is crazily unreadable, and really needs a comment. Of course, regular expressions in general tend to cause readability problems on their own; it's notable that my thought on writing this was to think "hey, we could just get rid of the regular expression entirely and see what happens"—what happens is that we start treating URIs with '.' in them, such as /ongoing/When/200x/2007/06/17/IMGP5702.png
, as pages, when they aren't, so all the results are wrong.
You can't do this easily by avoiding regular expressions entirely; Tim's URI layout means that /ongoing/When/200x/2007/06/17/
is an archive list page, rather than a genuine entry page, so you can't just check with a '.' somewhere in the URI (although this is actually slightly slower than using a regular expression anyway). However looking into this in detail brought up errors in the regular expression parsing as well: /ongoing/When/200x/2005/07/14/Atom-1.0
is a valid page URI, but the regex thinks it's an auxiliary file. There's also the unexpected /ongoing/When/200x/2005/04/18/Adobe-Macromedia?IWasEnlightenedBy=MossyBlog.com
, which while not strictly within Tim's URI layout, is allowed to exist and be a page resource by his server configuration. Regular expressions, although powerful, are very difficult to craft correctly; this is made much harder by the problem being (essentially) an ad hoc one: I doubt Tim ever sat down and designed his URI layout with a thought to doing this kind of processing on it. (Even if he had, it would probably get caught out by something similar.)
import re, sys, parallel
def top(dct, num=10):
keys = []
last = None
def sorter(k1,k2):
if k2==None:
return 1
diff = cmp(dct[k1], dct[k2])
if diff==0:
return cmp(k2,k1)
else:
return diff
for key in dct.keys():
if sorter(key, last)>0:
keys.append(key)
keys.sort(sorter)
if len(keys)>num:
keys = keys[1:]
last = keys[0]
keys.reverse()
return keys
hit_re = re.compile(r'^/ongoing/When/\d\d\dx/\d\d\d\d/\d\d/\d\d/[^ .]+$')
hit_re_search = hit_re.search
hit_str = "/ongoing/When/"
ref_str = '"http://www.tbray.org/ongoing/'
def report(label, hash, shrink = False):
print "Top %s:" % label
if shrink:
fmt = " %9.1fM: %s"
else:
fmt = " %10d: %s"
for key in top(hash):
if len(key) > 60:
pkey = key[0:60] + "..."
else:
pkey = key
if shrink:
print fmt % (hash[key] / 1024.0 / 1024.0, pkey)
else:
print fmt % (hash[key], pkey)
print
def processor(lines, driver):
u_hits = driver.get_accumulator()
u_bytes = driver.get_accumulator()
s404s = driver.get_accumulator()
clients = driver.get_accumulator()
refs = driver.get_accumulator()
def record(client, u, bytes, ref):
u_bytes[u] += bytes
if hit_str in u and hit_re_search(u):
u_hits[u] += 1
clients[client] += 1
if ref !='"-"' and not ref.startswith(ref_str):
refs[ref[1:-1]] += 1 # lose the quotes
for line in lines:
f = line.split()
if len(f)<11 or f[5]!='"GET':
continue
client, u, status, bytes, ref = f[0], f[6], f[8], f[9], f[10]
if status == '200':
try:
b = int(bytes)
except:
b = 0
record(client, u, b, ref)
elif status == '304':
record(client, u, 0, ref)
elif status == '404':
s404s[u] += 1
return [u_hits, u_bytes, s404s, clients, refs]
(u_hits, u_bytes, s404s, clients, refs) = parallel.process(sys.argv[1], processor)
print "%i resources, %i 404s, %i clients\n" % (len(u_hits), len(s404s), len(clients))
report('URIs by hit', u_hits)
report('URIs by bytes', u_bytes, True)
report('404s', s404s)
report('client addresses', clients)
report('referrers', refs)
Supporting library code (134 LOC)
Perhaps 30 LOC here is logging and debugging, or could be removed by getting ridding of a layer or two of abstraction (I had lots of different driver types while working on this). This is actually my final running code: note that various things aren't needed any more (such as the chunksize
parameter to ParallelDriver.process_chunk
). This impedes readability a little, but hopefully it's still fairly obvious what's going on: we run J
children, each of which subdivides its part of the data into a number of equal chunks (calculated based on the total memory we want to use, but calculated confusingly because I tried various different ways of doing things and evolved the code rather than, you know, thinking), and processes and reduces each one separately. The result per child gets pushed back over a pipe, and we run a final reduce per child in the parent process.
import os, mmap, string, cPickle, sys, collections, logging
logging.basicConfig(format="%(message)s")
class ParallelDriver:
# chunksize only used to detect overflow back to start of previous chunk
def process_chunk(self, processor, mm, worker_id, chunk_id, chunk_start, chunk_end, chunksize):
start = chunk_start
end = chunk_end
if start>0:
if mm[start]=='\n':
start -= 1
while mm[start]!='\n':
start -= 1
if mm[start]=='\n':
start += 1
# [start, end) ie don't include end, just like python slices
mm.seek(start)
class LineIter:
def __init__(self, mm):
self.mm = mm
def __iter__(self):
return self
def next(self):
c1 = self.mm.tell()
l = self.mm.readline()
c2 = self.mm.tell()
if c2 > end or c1 == c2:
raise StopIteration
return l
it = LineIter(mm)
result = processor(it, self)
return result
def get_accumulator(self):
return collections.defaultdict(int)
class ParallelMmapFilesMaxMemDriver(ParallelDriver):
def __init__(self, file):
self.file = file
def process(self, processor):
# based on <http://www.cs.ucsd.edu/~sorourke/wf.pl>
s = os.stat(self.file)
f = open(self.file)
j = os.environ.get('J', '8')
j = int(j)
maxmem = os.environ.get('MAXMEM', 24*1024*1024*1024)
maxmem = int(maxmem)
size = s.st_size
if maxmem > size:
maxmem = size
chunksize = maxmem / j
PAGE=16*1024
if chunksize > PAGE:
# round to pages
chunksize = PAGE * chunksize / PAGE
if chunksize < 1:
chunksize = 1
total_chunks = size / chunksize
chunks_per_worker = float(total_chunks) / j
commfiles = {}
for i in range(0, j):
commfile = os.tmpfile()
pid = os.fork()
if pid:
commfiles[pid] = commfile
else:
pickle = cPickle.Pickler(commfile)
result = None
worker_start = int(i*chunks_per_worker) * chunksize
worker_end = int((i+1)*chunks_per_worker) * chunksize
if i==j-1:
worker_end = size
chunks_for_this_worker = (worker_end - worker_start) / chunksize
for chunk in range(0, chunks_for_this_worker):
chunk_start = worker_start + chunk*chunksize
if chunk_start >= size:
break
chunk_end = worker_start + (chunk + 1)*chunksize
if chunk==chunks_for_this_worker-1:
chunk_end = worker_end
mm = mmap.mmap(f.fileno(), size, mmap.MAP_SHARED, mmap.PROT_READ)
interim_result = self.process_chunk(processor, mm, i, chunk, chunk_start, chunk_end, chunksize)
mm.close()
if interim_result==None:
continue
if result==None:
result = interim_result
else:
for idx in range(0, len(interim_result)):
for key in interim_result[idx].keys():
result[idx][key] += interim_result[idx][key]
pickle.dump(result)
commfile.close()
sys.exit(0)
final = None
for i in range(0, j):
(pid, status) = os.wait()
readf = commfiles[pid]
readf.seek(0)
unpickle = cPickle.Unpickler(readf)
result = unpickle.load()
if result==None:
readf.close()
continue
if final==None:
# first time through, set up final accumulators
final = []
for i in range(0, len(result)):
final.append(self.get_accumulator())
for i in range(0, len(result)):
for key in result[i].keys():
final[i][key] += result[i][key]
readf.close()
f.close()
return final
def process(file, processor):
d = ParallelMmapFilesMaxMemDriver(file)
return d.process(processor)
Final thoughts
First off: functional languages. Let's use them more. Mauricio Fernandez's OCaml implementation is still the leader, and despite being a little more verbose than the Ruby original is still pretty damn readable (he's actually just announced an even faster one: 25% faster by using block rather than line-oriented I/O; the LOC count is creeping up with each optimisation he does, though). Functional languages require you to think in a different way, but when you're dealing with streams of data, why on earth would you not want to think like this? Better, OCaml gives you imperative and object-oriented language features as well, and seems to pack a solid standard library. I haven't learned a new language for a while, and I'm a little rusty on the functional languages I've been exposed to anyway; I guess OCaml is going to be my next.
Second off: compilers. Let's use them more as well. It's all very well to have an interpreted language where you have no build phase, and can just dive straight into fiddling with code, but I'm really not convinced this is a valid optimisation of the programming process. For one thing, if you're working with tests (and please, work with tests), my experience is that running them will vastly out-weigh any compile time; in particular, if you use an interpreted language, it is likely to completely mask the compilation time. There are enough good, high-level, compiled languages around that compilation doesn't put you on the wrong end of an abstraction trade-off (the way using assembler over C used to before everyone gave up writing assembler by hand).
I have an anecdote that I think further backs up my assertion that a compilation step isn't an impediment to programming. The only time I can remember writing a non-trivial program and having it work first time was with a compiled language. Not because of type safety, not because the compiler caught lots of foolish mistakes. What happened was I was using voice recognition software to control my computer, due to a very serious bout of RSI, so I spent a fair amount of time thinking about the problem before going near the computer. It took about the same amount of time overall as if I'd been able to type, and had started programming straight away—a couple of days total. But this way, I wasn't exploring the problem at the computer, just trying things out in the way I might with an interpreted language, particularly one with an interpreter shell. Programming is primarily about thinking: in many cases doing the thinking up front will take at most as long as sitting down and starting typing. Plus, you can do it at a whiteboard, which means you're standing up and so probably being more healthy, and you can also pretend you're on House, doing differential diagnosis of your problem space.
There are situations where you want to explore, of course; not where you have no idea how to shape your solution, but rather where you aren't sure about the behaviour of the systems you're building on. WF-2 has been mostly like that:
much of my time was actually spent writing subtle variants of the parallel driver to test against each other, because I don't understand the effects of the T1 processor, ZFS, and so on, well enough. A lot of the remaining time was spent in debugging line splitting code, due to lack of up-front thought. Neither of which, as far as I'm concerned, is really getting to the point of WF-2. I suspect this is true of most people working on WF-2; you choose your approach, and it comes down to how fast your language goes, how smart you are, and how much time you spend profiling and optimising.
Anyway, you need to have a rapid prototyping environment to explore the systems you're working with, perhaps an interpreted language or one with a shell; and you want a final implementation environment, which may want to use a compiled language. For the best of both worlds, use a language with an interpreter and a native code compiler. (Perhaps unsurprisingly, OCaml has both.)
Where does this leave us with WF-2? None of my original three questions has been answered, although I certainly have learned other things by actually getting my hands dirty. It may be premature to call this, because people are still working on the problem, but I'm not aware of anyone trying a different approach, architecturally speaking, probably because straightforward data decomposition has got so close to the theoretical best case. The optimisations that we've seen work at this scale are helpful—but may not be trivial for the programmer-on-the-street to use, either because language support is sometimes flimsy, or because they'll be building on top of other people's libraries and frameworks. So it's good news that, besides automatic parallelisation of user code, it's possible to parallelise various common algorithms. GCC has parallel versions of various algorithms, there are various parallel libraries for Java, and we can expect other systems to follow. For obvious reasons there's lots of research in this space; check out a list of books on parallel algorithms. In particular check out the dates: this stuff has been around for a long time, waiting for us normal programmers to need it.
- Published at
- Thursday 12th June, 2008
- Tagged as
- Scaling
- Widefinder
- Parallelism
So far I've found some interesting ways to suck in Widefinder. Let's find some more.
Analysing the lifecycle
I didn't really design the lifecycle of my processes; I was hoping I could just wing it by copying someone else and not think about it too much. Of course, when translating between languages, this isn't a great idea, because things behave differently; and not taking into account the new, enormous, size of the data set was a problem as well. 42 minutes isn't a terrible time, and I suspect that Python will have difficulty matching the 8-10 minutes of the top results, but we can certainly do better.
An obvious thing to do at this point is to look at how long the master, and each worker, actually spends doing the various tasks. What happens is that the master maps the file into memory, then forks the workers, each of which goes over the lines in its part of the file (with a little bit of subtlety to cope with lines that cross the boundaries), then serialises the results back over a pipe using cPickle
. The master waits on each worker in turn, adding the partial results into the whole lot; then these results get run over to generate the elite sets for the output.
The following is taken from a run over the 10 million line subset. Other than providing logging to support this display, and removing the final output (but not the calculation), there were no other changes to the code.
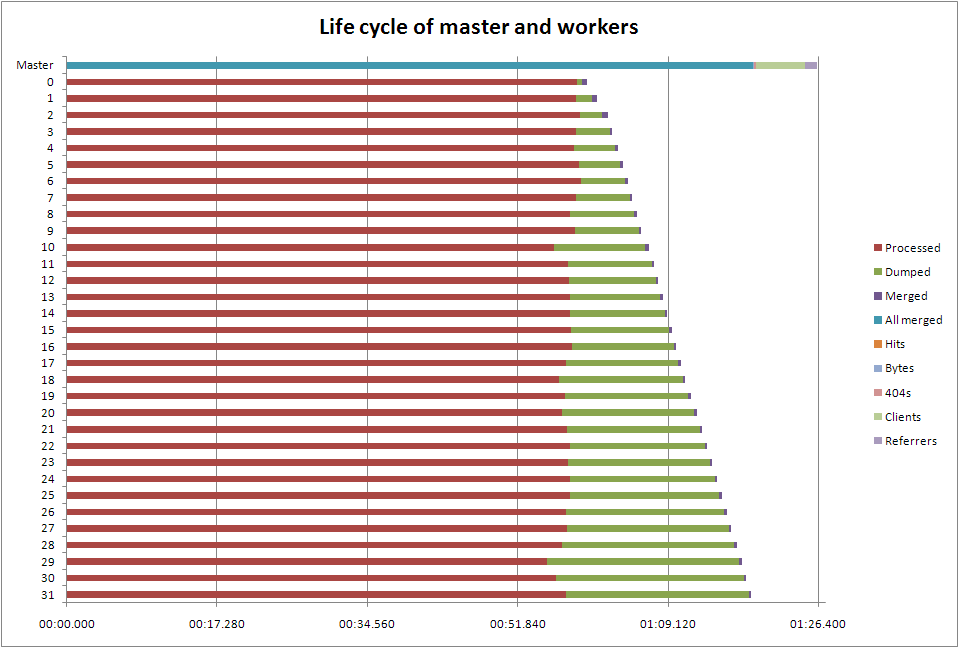
One thing immediately jumps out: because the workers aren't guaranteed to finish in the order we started them, we're wasting time by having the master process them in order. The time between the first worker to finish and the one we started first to finish is more than 20 seconds; in the full run we process about 20 times more data, so we'd expect that gap to become much larger. Out of order processing would seem to be worth looking at
It takes about half a second to dump, and another half a second to undump, data between each worker and the master: that's 30 seconds on this run, so again we might expect a significant penalty here. In another language we might try threads instead of processes, but Python isn't great at that. Alex Morega moved to temporary files using pickle, and saw an improvement over using whatever IPC pprocess uses. Temporary files would be worth investigating here; however it seems unlikely I can find a faster way of dumping my data than using cPickle
.
Merging takes about 0.2s per worker, which isn't terribly significant. However the client and referrer reports both take significant time to process: nearly six seconds for the client report. It's been pointed out by Mauricio Fernandez that the current elite set implementation is O(nm log m)
(choosing the m
top of n
items), on top of the at-best O(n)
merges (the merges depend on the implementation of dictionaries in Python). Mauricio came up with a method he says is O(n log m)
, which would be worth looking at, although with m=10
as we have here, it won't make much difference.
You can't see it on this chart, but it looks like the line by line processing speed is constant across the run, which is really good news in scaling terms.
Other things to look at
There's the memory problem from before, where I could either run twice as many workers, in two batches (or overlapped, which should be more efficient), or I could make each worker split its workload down into smaller chunks, and memory map and release each one explicitly. The latter is easier to do, and also doesn't carry the impact of more workers.
Since on the T2000 the 32 bit versions of Python run faster than the 64 bit versions, making it so that each mapped chunk is under 2G would allow me to use the faster interpreter. That will actually fall out of the previous point, in this case.
I'm currently using a class I wrote that wraps the Python built-in dict
; I should be able to extend it instead, and possibly take advantage of a Python 2.5 feature I was unaware of: __missing__
. That's going to affect the processing of every single line, so even if the gains are slight, the total impact should be worthwhile.
In WF-1, Fredrik Lundh came up with some useful optimisations for Python. I'm already compiling my regular expressions, but I should roll in the others.
Tim's now installed the latest and greatest Sun compiler suite, so rebuilding Python 2.5 again with that might yield further speed improvements. He's also installed a SPARC-optimised Python 2.5, so perhaps that's already as fast as we're getting.
Other parallelisms
I missed WF 1 in LINQ the first time round, which mentioned PLINQ. The CTP (community technology preview) for this came out earlier this month, and Jon Skeet has been playing with it. PLINQ is exactly what I was talking about at the beginning of this series.
- Published at
- Tuesday 10th June, 2008
- Tagged as
- Scaling
- Widefinder
- Parallelism
After yesterday's fun with pretty graphs, I did some investigation into what the system was actually doing during my runs. I noticed a number of things.
Before doing any of this, I did a run with the full set of data: 2492.9 seconds, or about 41 minutes. I was hoping for something in the range 35-45 minutes, based on a rough approximation, so as a first stab this isn't too bad. It's worth re-iterating though, that this is still an order of magnitude worse than the leading contenders, so the big question is whether this remains just an optimisation problem for me, or if there are other things impeding parallelism as well. Anyway, on to my observations.
- I was generally using each CPU about a third to half as much as is possible.
- My drive throughput was between 7M/s and 20M/s per drive. Presumably I'm processing somewhere in between, so we're seeing read-ahead buffering do its thing; however I'm still not able to keep the drives operating at a consistent throughput, let alone at their maximum, which is more than double that.
- I'm being very naive about resource usage at the moment.
- When I did a run over the complete 42G of data, I ended up having 42G of RSS.
- A parallel read of all the data, not even processing it, took 100 times as long as a parallel read of 10m lines
The first I've noticed during other people's runs as well. With me, it isn't in disk stalls, because I'm not seeing significant asvc_t
against the two drives behind the ZFS volume. However the patterns of asvc_t
against drive utilisation (%b
in iostat -x
) vary for different implementations. This all suggests to me that there are gains to be had by matching the implementation strategy more closely to the behaviour of the I/O system, which isn't really news, but might have more impact than I expected. Of course, for all I know, the best implementations are already doing this; it would be nice to know what about them is better, though, and whether it's applicable beyond this setup, this data, this problem.
Anyway, what do we get from this? That I've failed to observe my first rule in looking at any scaling problem: making sure we don't run out of memory.
Memory
If you use mmap
to map a file larger than core, and then read through the entire file, you end up with the whole thing mapped into core. This doesn't work, of course, so you get slower and slower until you're swapping like crazy; this of course means you get process stalls, and the ever-helpful operating system starts doing more context switches. So the entire thing melts: it's fun. This is still true even if we split the file across multiple processes; elementary arithmetic should tell us that much, but hey, I went ahead and demonstrated it anyway, even if that's not what I meant to do.
So what I really should do instead is to do multiple runs of J processes at a time; enough runs that the total amount mapped at any one time will always be less than available memory. Another approach might be to munmap
chunks as you go through them; I actually prefer that, but I don't think it's possible in Python.
I'm hoping this is why we suddenly go crazy between processing 10m lines and all of them.

The slope between 100k and 10m is around seven; between 10m and the whole lot it's about 30. However the slopes lower down are a lot lower than seven, so there's something else going on if they're representative (they may be not simply because the data is too small). If they are, there's a pretty good fit curve, which is very bad news for the scalability of what I've written.
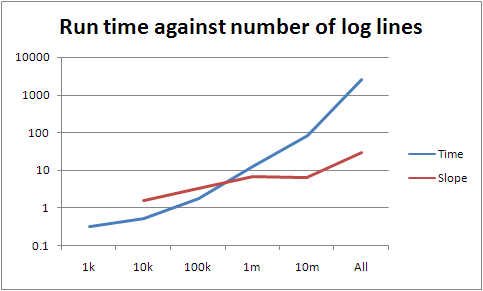
The obvious thing that is changing as we get bigger (beyond running out of memory) is the size of the partial result sets. These have to be serialised, transferred, unserialised, reduced, lost, found, recycled as firelighters, and finally filtered into an elite set. The bigger they are, the longer it takes, of course, but something much worse can go wrong with a map with many items: it can bust the assumptions of the underlying data structure. I don't think that's happening here, as we only have a few million entries in the largest map, but it's something that's worth paying attention to when thinking about scaling. (I have some thoughts related to this at some point, although you could just read Knuth and be done with it. Sorry, read Knuth and about five hundred papers since then and be done with it. Oh, and you have to know what data structures and algorithms are being used by your underlying platform.)
Anyway, today I've mostly been proving how much I suck. I have some other stuff coming up; I've gathered a fair amount of data, and am still doing so, but it's getting more fiddly to either analyse or to pull this stuff into graphs and charts, so that's it for today. Expect something in the next couple of days.
- Published at
- Monday 9th June, 2008
- Tagged as
- Scaling
- Widefinder
- Parallelism
Last year, Tim Bray ran a mini-investigation, based on the idea of parallelising traditionally linear tasks. He wrote a simple web logfile analyser, without any fancy tricks, and watched other people beat its performance in various ways, providing colour commentary along the way. (Should that be color commentary, given that we don't actually have that phrase in British English?). He called it Wide Finder, and the results, although somewhat unscientific because of the constraints he was under, showed the best performance in terms of elapsed time on a multicore T5120 some three orders of magnitude better than Tim's linear implementation in Ruby, with about an order of magnitude more code. The T5120, as Tim pointed out, is the shape of the future, both in the data centre and on the desktop; it doesn't matter who your processor designer of choice is, these things are in scale out rather than scale up mode for at least the next few years.
Now, he wants to do it again, only better: Wide Finder 2 gives people the opportunity to write their own faster version of Tim's linear code in whatever way they want, and to run it on a T2000. Tim is concerned with a balance of complexity and performance; complexity is mainly being measured in LOC, which is probably reasonable to get things going. The crucial idea is that we need techniques for taking advantage of modern multi-core and multi-thread techniques in processors that don't require everyone to be experts in concurrency and multiprocessing. This could prove interesting.
There are three things that I think we should try to shed some light on after WF-1. If you look back over the results, several use memory mapping to reduce the I/O overhead as much as possible, and then have multiple workers go over the space of the file, in chunks, either with OS threads or processes as workers, or something managed by the VM of the language itself. Either you have lots of little chunks, or you have as many chunks as workers, which I'd guess is less strain on the data pre-fetch scheduler in the operating system. Whatever, we're talking about data decomposition with independent data (as in: the processing of each individual log line is independent of other log lines or the results of their processing). This is the easiest kind of data decomposition. So: three things we can investigate from here.
- Can we do data decomposition in the independent data case automatically?
- For the kinds of problems that we know data decomposition with independent data works well, can we come up with better approaches?
- Are there reasonable kinds of problems for which data decomposition is either much too complicated or simply not applicable?
I'll tackle my thoughts on each of them separately, in that order. Most of the stuff I've done is on the first one, as this seems the most interesting to me.
Can we do data decomposition in the independent data case automatically?
There are two ways I can think of for doing this, one of which isn't strictly automatic but is more applicable. This is assuming that all your data comes from the same place to start off with, and that everything's running on one machine, although I'm pretty sure both of those could be lifted with a bit of cleverness (the setup becomes harder, but the user code should remain the same).
Cheating
The first way is kind of cheating. Assume we're dealing with a single loop over the data in the linear case, ie a single reduction. If you write your loop using a line iterator or generator, you can put it all in a function which takes a line generator and some sort of accumulator; a library can then drive your function and take care of making it work in parallel. Let's work through an example in Python to see how this might work. I'm not going to do the WF-2 example like this yet, because it's close to 100 lines long. Let's just calculate the arithmetic mean of a list of integers. This is pretty easy to do in the linear style.
import sys
f = open(sys.argv[1])
n = 0; s = 0
for line in f.readlines():
try:
i = int(line)
s += i
n += 1
except:
# ignore invalid lines
pass
print (float(s) / n)
So we change things around so we're not driving the loop any more.
import sys, parallel
def processor(lines, driver):
acc = driver.get_accumulator()
for line in lines:
try:
i = int(line)
acc.acc('s', i)
acc.acc('n', 1)
except:
pass
return acc
result = parallel.process(sys.argv[1], processor)
print (float(result['s']) / result['n'])
This is very similar in terms of both plain LOC and complexity, although of course there's stuff hiding in the parallel
module. For a linear implementation, that's another 50 odd lines of code; for a fairly simple parallel implementation using mmap
and a pipe-fork approach, it's over 100. It's tedious code, and not as efficient as it could be; my aim isn't to build this for real, but to nudge towards an existence proof (really an existence hunch, I guess). I won't bother showing it here for that reason, and because the point is that it's infrastructure: it gets written it once so the user code doesn't have to worry about it. Mine is basically Sean O'Rourke's WF-1 winner, translated to Python.
Forking a load of children costs time, moving partial results around and accumulating them takes time, and that can overwhelm the advantages of running on multiple cores when you don't have a large data set; for the simple arithmetic mean, you have to have larger files than I could be bothered with to show an improvement; doing a similar job of counting Unix mbox From_
lines, the parallel version across eight cores was about three times better than the linear version. I haven't bothered trying to optimise at all, so it's likely I'm doing something dumb somewhere.
import sys, parallel
def processor(lines, driver):
acc = driver.get_accumulator()
for line in lines:
try:
if line[0:5]=='From ':
acc.acc('n', 1)
except:
pass
return acc
result = parallel.process(sys.argv[1], processor)
print result['n']
Some empirical data, for the Unix mbox job: running on a machine with dual quad-core 1.6GHz Xeons, averaging over 10 consecutive runs from warm (so the roughly 1GB file should already have been in memory somewhere), we get the following. (Note that the GNU grep(1)
does it in a little more than two seconds; this is always going to be a toy example, so don't read too much into it.)
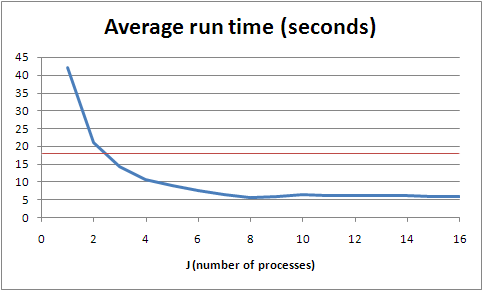
The red line is the mark for my naive linear implementation; its main advantage is that a lot of the work is being done in the interpreter, in C, rather than in my code, in Python. This makes a big difference - the parallel version seems to be doing about 2.5 times as much work. It's worth noting that this machine is not completely unloaded; it's multi-user and also acts as a web and mail server, amongst other things, so beyond J=6 we're seeing slightly more flaky numbers. Back-of-the-envelope monitoring, however suggests that we don't start getting interference from other processes on the box before we start seeing interference from its own processes, where J=9 and we run out of cores (although the system does a reasonably good job of keeping things going from there up).
Note that there's some interesting discussion going on around WF-2 about how to scale some of these techniques up to gigabytes of input in one go; Alex Morega in particular has been using a broadly similar approach in Python and hit some interesting snags, and I urge you to read his write-up for details. Either we'll come up with new idioms that don't have these problems, or we'll improve the things we depend on (languages, libraries, VMs, operating systems...) to allow us to work better at scale without radically changing the way we code. (I'm sure there are some great approaches to these problems that I haven't heard of - with luck, WF-2 will bring them to a wider group of people.)
Enough on this. Hopefully I've convinced you that this is entirely feasible; it's a matter of someone writing a good library to drive it all.
Not cheating
In order to be more automatic, we need to convert the original linear style into the parallel style entirely programmatically. To my knowledge, you can't do this in Python. In fact, many languages, even dynamic languages, don't want you dicking around directly with live code constructs, which limits the applicability of this idea somewhat. However it's entirely possible to imagine that we could, in some theoretical super-Python, write the following and have the parallel
module rewrite the process
function appropriately.
import sys, parallel
def process(file):
f = open(sys.argv[1])
result = { 'n': 0, 's': 0 }
for line in f.readlines():
try:
i = int(line)
result['s'] += i
result['n'] += 1
except:
pass
f.close()
print (float(result['s']) / result['n'])
parallel.apply(process, sys.argv[1])
In complexity for the programmer, this is like a halfway house between the linear and parallel approaches above, with the nice advantage that it doesn't remotely look parallel except for the last line.
I'm certain it is possible because the only things that enter the loop either can be considered immutable (in the scope of the loop), or are the file object f
(which we only ever apply readlines()
to), and the only thing that escapes the loop is a dictionary, and that within the loop we only ever accumulate into that dictionary. It's not beyond the bounds of programming ability to spot this situation, and convert it into the cheating version above. Although it's probably beyond my programming ability, and certainly is in Python. In fact, we could probably write a parallel.invoke()
or something which is given a Python module, and parallelises that, at which point we've got a (limited) automatic parallel Python interpreter. Again, providing you can mutate Python code on the fly.
A question which arises, then, is this: given the constraints of only being able to parallelise loops over quanta of data (for instance, iterating over log lines, or 32 bit words, in a file), with immutable inputs and a number of dictionary outputs, how large is the problem space we can solve? This is actually question three on the original list, so I'll leave it for now.
Another is whether we can lift any of these restrictions, the main one being accumulation. (And I'm counting subtraction as accumulation, for these purposes.) Assuming data independence, there aren't actually many alternatives to accumulation: I can only think of multiplication, which is the same as accumulation anyway, give or take some logarithms. So I'm guessing the answer to this question is an unwelcome "no"; however I'm probably wrong about that. You can do an awful lot with accumulation, though.
For the kinds of problems that we know data decomposition with independent data works well, can we come up with better approaches?
I'll be very disappointed if the answer to this isn't "yes". However I don't think WF-2 is necessarily going to show us much here by being almost tuned to this kind of approach. I'm probably wrong about this as well, though, and new ways of thinking about these kinds of problem would be great.
It's not clear to me, because I don't understand the languages well enough, whether all the techniques that were used in WF-1 with JoCaml and Erlang are covered by data decomposition (beyond things like optimising the matcher). Even if there aren't, there are undoubtedly lessons to be learned from how you structure your code in those languages to approach these problems. This partly falls under the previous question: if we can't automatically parallelise our old ways of doing things, then we want new idioms where we can.
Are there reasonable kinds of problems for which data decomposition is either much too complicated or simply not applicable?
The simple answer is "yes", but that's in general. WF-2 is concerned with what most programmers will have to deal with. Here, I wonder if the answer might be "no". You can do an awful lot without sacrificing data independence. I calculated the arithmetic mean earlier, but for instance you can do standard deviation as well, providing you defer most of the computation until the end, and are prepared to unwind the formula. Generally speaking, we don't seem to actually do much computation in computing, at least these days. I think this means that for most people, beyond this one technique, you don't have to worry about parallelism too much at the moment, because anything that isn't linear to start off with (like, say, web serving) is already scaling pretty well on modern processors. So if we can use data decomposition automatically in some common cases, we dodge the bullet and keep up with Moore's Law for a few more years.
Dependent data decomposition
Data decomposition continues to be useful even with related data; however you start getting into I/O or memory model issues if your data set starts off all jumbled up, as it is in WF-2, because you've got to sort it all to get it into the right worker. For instance, if you want to do logfile analysis and care about sessions, it becomes difficult to do anything without arranging for all the data within a session to go to the same worker. (Not impossible, but in the context of making it easy on programmers, I think we can ignore that case.) In most cases, you're better off filtering your data once into the right buckets, ideally at the point you collect it; you can of course parallelise filtering, but if you're doing that at the same time as your final processing, you're moving lots of data around. The only situation I can think of where it's not going to help to filter in advance is if you need to do different processing runs over the data and your dependencies in the processing runs are different, resulting in a different data decomposition. On the other hand, I can't think of a good example for this. I'm sure they exist, but I'm less sure that they appear to regular programmers.
Note that in my parallel
module above, for dealing with an independent data problem, I made a silent assumption that it's cheaper to not move much data around between the parts of your system; so it's far better for the controlling part of the system to tell a worker to attack a given byte range than it is to actually give it that data. This is surely not true in the general case, which is great news for dependent data decomposition. Given suitable VM infrastructure, or an appropriate memory model, this shouldn't actually be a problem; it's just that for most languages on most platforms at the moment, it seems to be. On the other hand, once you scale beyond a single machine, you really want the data you're working on to be close to the processing; a large part of the magic of Hadoop seems to be about this, although I haven't looked closely.
First results for WF-2
First results for WF-2 are beginning to come in. Ray Waldin set the bar using Scala, taking Tim's thousands of minutes down to tens. By now the leaders are running in minutes - note that if the ZFS pool can pull data at 150Mbps, as the Bonnie run showed with a 100G test, then the fastest all the data can come off disk is a little under five minutes; we're seeing results close to that already.
I'll start posting timings from my runs once I get it up to the complete data set; I'm also looking at how the use efficiency changes by number of workers across the size of input file, since Tim has conveniently provided various sizes of live data. So this might take a few days; and there's a chance my times will be embarrassingly bad, meaning I might just not publish :-)
A final point
There's a great book called Patterns For Parallel Programming, by Mattson, Sanders and Massingill (if you're not in Europe you may prefer to get it from Amazon.com). It has a lot more detail and useful thoughts on parallelism than I could ever come up with: although I have some experience with scaling and data processing, these guys do it all the time.